At present, there are more than 110000 code vulnerabilities included in CVE. In the process of program development, the existing vulnerable code snippets are often reused. We start with binary programs testing, using data mining and deep learning technology to train feature models from the perspectives of syntax and semantics, which could effectively detect vulnerability code reuse, malicious code and predict high-risk code in programs. We developed Vulseeker-pro, a vulnerability search tool for cross-platform binaries, which achieves more accuracy and efficiency than Berkerly Gemini and other tools.
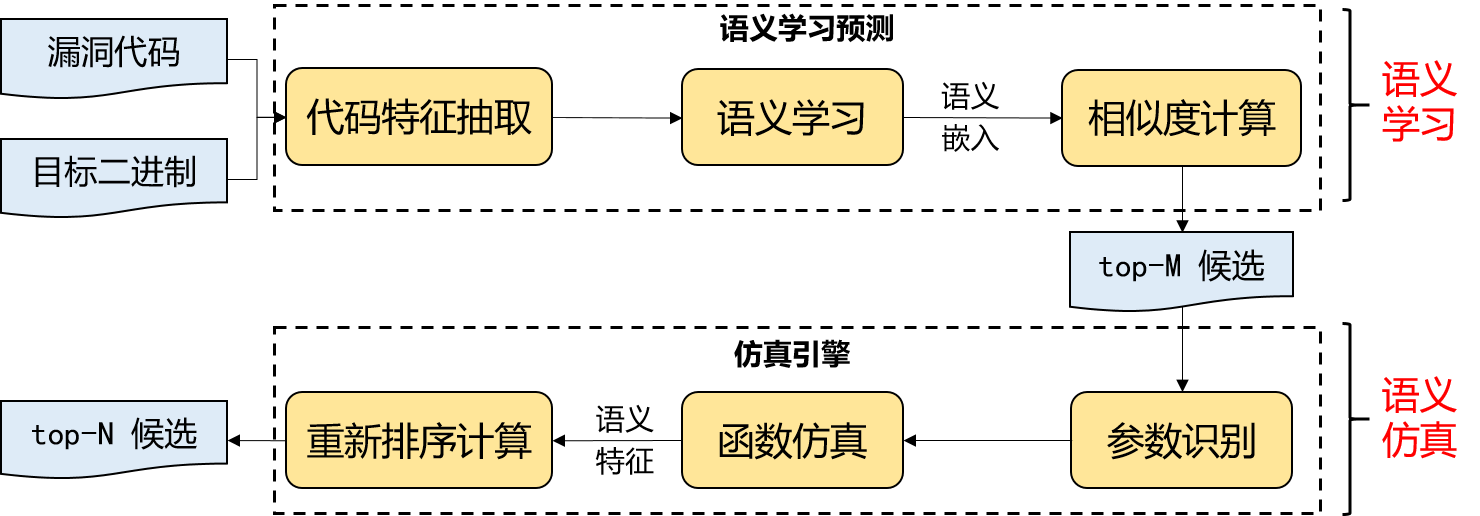
Related Papers:
- Jian Gao, Xin Yang, Ying Fu, Yu Jiang, Heyuan Shi, and Jiaguang Sun:VulSeeker-pro: enhanced semantic learning based binary vulnerability seeker with emulation.(ESEC/FSE 2018: 803-808)
- Jian Gao, Xin Yang, Ying Fu, Yu Jiang, and Jiaguang Sun: VulSeeker: a semantic learning based vulnerability seeker for cross-platform binary.(ASE 2018: 896-899)
See Vulseeker-pro through:https://github.com/buptsseGJ/VulSeeker
In the fuzz testing for memory security, we focus on solving the challenges of the source and mutation convergence of seeds. We proposed a seed generation method based on symbol execution, and then developed a directed mutation algorithm derived from coverage information. Through directed mutation, the scheduling strategy is optimized to increase the number of mutations reaching the rare code path and ensure that the branching conditions on the path will not be destroyed. Our work has achieved higher coverage than the widely used mainstream fuzz testing tools such as Google libfuzzer AFL.
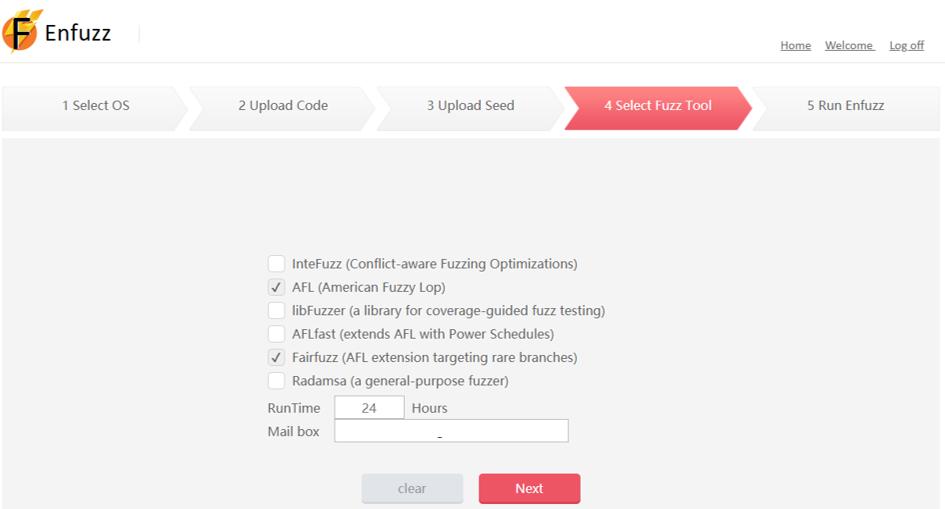
Related Papers:
- Mingzhe Wang, Jie Liang, Yuanliang Chen, Yu Jiang, Xun Jiao, Han Liu, Xibin Zhao, Jiaguang Sun:SAFL: increasing and accelerating testing coverage with symbolic execution and guided fuzzing. (ICSE (Companion Volume) 2018: 61-64)
- Jie Liang, Yu Jiang, Yuanliang Chen, Mingzhe Wang, Chijin Zhou and Jiaguang Sun: PAFL: Extend Fuzzing Optimizations of Single Mode to Industrial Parallel Mode. (ESEC/SIGSOFT FSE 2018: 809-814)
See the software through:http://wingtecher.com/Enfuzz
Nowadays, the functions of Android applications are becoming more and more complex. The privacy and security information involved are becoming more and more in-depth, and the implementation methods are becoming more and more diverse, which puts forward higher demand for the automatic test case generation tool for Android applications. To meet the test requirements of industrial complex applications, we comprehensively considered various factors include test history and test intensity, and implemented a dynamic weight algorithm guided by the growth of code coverage. The algorithm can effectively generate event sequences to trigger deep pages of applications. The tool adopts C / S architecture, supports simultaneous testing of multiple devices and tasks, and shares application model data and test progress data between devices and tasks. It has achieved better results than existing tools or algorithms such as Monkey、Priority Set in improving the testing coverage of the tested application.
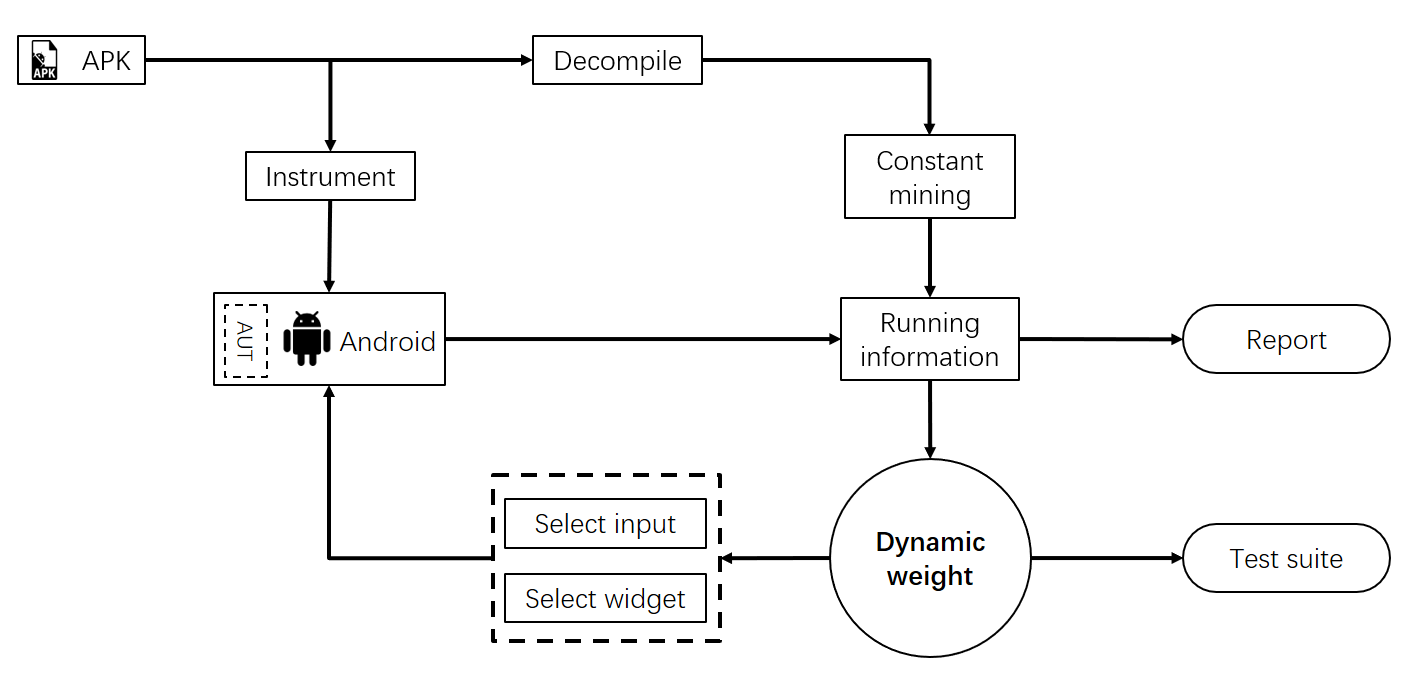
Today, deep learning has been widely adopted in security-related fields, such as unmanned driving and malware detection, which brings new challenges to the wide application of deep learning technology. However, deep learning systems often exhibit incorrect behavior in boundary cases. We proposed a deep learning system testing tool DLFuzz, which combines fuzz testing techniques with deep learning testing techniques to generate adversarial samples by adding small perturbations to the test images to mislead the network. DLFuzz is able to generate more adversarial samples in less time with less perturbation than DeepXplore, and improve neuron coverage at the same time.
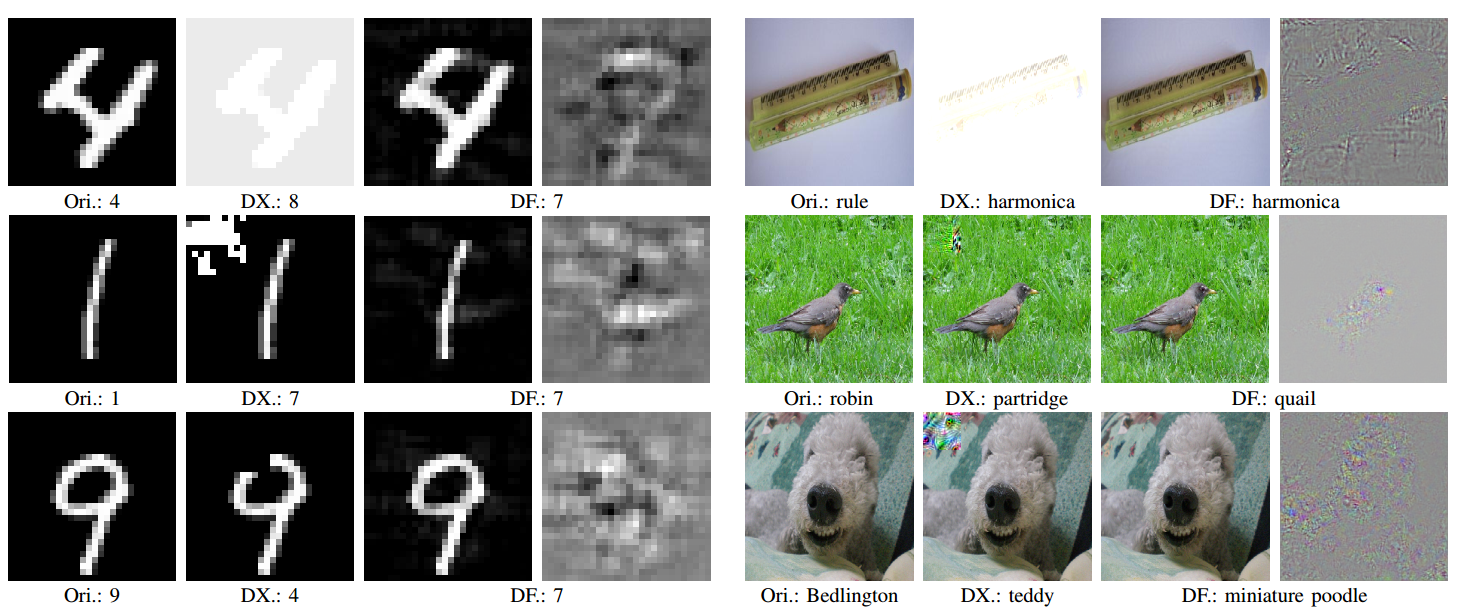
Related Papers:
- Jianmin Guo, Yu Jiang, Yue Zhao, Quan Chen, Jiaguang Sun:DLFuzz: Differential Fuzzing Testing of Deep Learning Systems. (ESEC/SIGSOFT FSE 2018: 739-743)
See DLFuzz through:https://github.com/turned2670/DLFuzz